Abstract
Innovation and technology are transforming peer review, with artificial intelligence (AI) and automation streamlining tasks, such as plagiarism detection, reviewer selection, formatting, and statistical checking, and significantly boosting efficiency. Yet, concerns around bias, data security, and the potential reduction in human oversight remain central. Additionally, open and virtual peer review practices have been examined for their role in promoting transparency, though they introduce challenges like depersonalization, which can reduce the human element in the review process. Overall, the discussions in this article emphasize the importance of balancing technological advancements with human expertise to uphold fairness and quality in peer review.
Introduction
The Asian Council of Science Editors (ACSE) hosted an exclusive interview series featuring industry experts who shared insights, ideas, and perspectives on the technology transforming the peer review process (Figure 1). The discussions highlighted critical areas, such as AI-driven automation and open peer review, along with the challenges and opportunities these innovations bring to academic publishing.
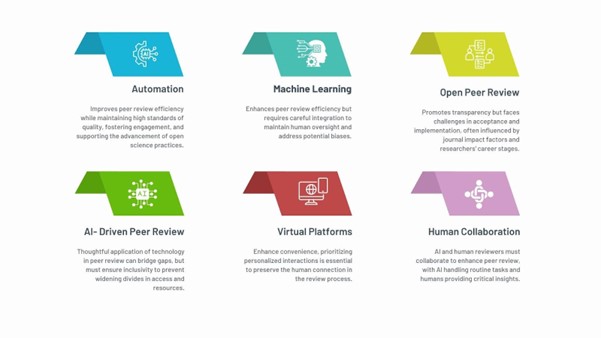
Open Peer Review: Transparency or Compromise?
A strong advocacy for open peer review, in terms of reviewer identity and comment openness, has been maintained, particularly as this mode of peer review has been widely practiced in the field for more than 20 year. However, it is less frequently accepted or utilized (negatively correlated), with the impact factor of the journal in question, as well as with the stage of the researcher’s (peer reviewer’s) career. Although reviewers are generally receptive to the idea of publishing their comments openly and with their names included alongside the articles they reviewed, there is a common reluctance to share feedback if their recommendation for an article is not accepted.1-3
An example of what likely is a common experience for early-career researchers can be found at Nature Communications, which has an open peer review process where reviewers can sign their reviews. A reviewer was invited to review an article authored by a senior colleague in the field based in the United State; someone with whom it was important to maintain a positive relationship. However, the quality of the paper was not particularly strong, raising a dilemma. Should participation in an open peer review process be accepted, potentially jeopardizing the relationship with this colleague? Ultimately, a compromise was made and the reviewer decided to opt out.4,5
How can journals and publishers manage these competing interests: the need to be open and transparent with peer review and therefore potentially speed up the process (e.g., article transfers/cascades between journals) and make it more ethical and compliant with ever-increasing standards in research ethics, while at the same time, balancing the needs of researchers (e.g., maintain reputations, relationships, etc.)? At present, there seems to be no universal solution. The pressure remains on researchers globally to publish in journals with the highest possible impact factors,6 and until this “model for recognition” changes, the widespread adoption of open peer review is likely to remain limited to the lower-tier journals and/or “mega-journals” or on preprint platforms.
Automation in Peer Review: Enhancing Efficiency or Risking Quality?
AI can help increase reproducibility, automate literature collection and analysis for systematic and umbrella reviews, and provide new analysis of existing data geared toward policy developments.7-9 These capabilities improve efficiency and contribute to policy development by providing fresh insights from existing data. Researchers acknowledge these advantages in their work. Thus, improving the review process through AI could make the activity faster and more enjoyable.
Automated scoring using text recognition would help diminish the volume of low-quality research that progresses through to peer review.1,10-12 This would enhance the overall quality of published work, as only the most rigorously vetted studies would reach publication. Linking reviews with reputation and career advancement will have the most significant impact on motivation. Providing reviewers with learning opportunities, recognition, and certification will undoubtedly increase their engagement and willingness to take on this kind of work. Moreover, mentorship programs could further ensure that the skills gained through peer review are transferable to other professional roles that academics often assume. This would benefit everyone, as continued engagement would enable reviewers to focus on critical analysis and creative insights.
Looking ahead, making peer review more equitable, integrative, and accessible could demystify the process and promote the adoption of open science practices.
Machine Learning in Peer Review: Game Changer or Double-Edged Sword?
Machine learning (ML) is a subfield of AI that uses algorithms trained on data to produce adaptable models capable of performing various complex tasks. ML has the potential to enhance efficiency by streamlining editorial triage and identifying appropriate reviewers, potentially increasing diversity and reducing reviewer fatigue.10 Additionally, it alleviates mundane tasks, minimizing human biases in reviewing specific research topics or institutions.1 However, despite its potential, ML has yet to reach its full capabilities. Many researchers remain cautious due to the nascent and rapidly evolving nature of the technology. Despite its potential, integrating ML into peer review carries risks. Key concerns include biased training data, which may inadvertently favor widely held ideas and specific regions that publish more frequently. Furthermore, reviewers bring valuable context from their experiences, including failed experiments and grant rejections, that ML might overlook. Worries also persist about the accuracy of AI-generated outputs and potential data privacy issues, particularly with sensitive unpublished work. Environmental concerns related to AI’s energy and water consumption raise questions, especially for publishers committed to sustainability goals.
Researchers are increasingly open to ML but remain wary of losing the human element in evaluations. While ML tools can combat reviewer fatigue and allow human reviewers to focus more on scientific merits, they risk oversimplifying complex assessments.10 To balance these benefits with risks, the industry needs clear, transparent, and standardized guidelines for AI use, coupled with robust data security measures and independent validation of ML models. The theme of innovation and technology in peer review highlights the urgency of finding new models to address current challenges. By leveraging ML, we can work toward a more efficient, transparent system, ensuring that reviewers continue to focus on the core task of evaluating science.
AI-Driven Peer Review: Objectivity or Bias?
AI-driven peer review offers both advantages and challenges when it comes to objectivity. On the positive side, AI can efficiently analyze submissions, detect plagiarism, and help select suitable reviewers, streamlining the process. However, its ability to be truly objective depends on the quality of the data used to train it. If that data is biased, the AI may unintentionally reinforce those biases.7,8 This can lead to underrepresenting certain regions or research topics.
To avoid these issues, human oversight is essential. Although AI can handle repetitive tasks, it cannot replace human reviewers’ critical thinking and judgment. Rigorous auditing of AI systems and databases is crucial to ensure fairness in the review process. Though AI has the potential to improve objectivity, the key is using it responsibly, ensuring that humans remain involved to balance the strengths and weaknesses.
Technology in Peer Review: Bridging Gaps or Widening Divides?
Technology has undoubtedly changed peer review, but whether it bridges gaps or creates new ones depends on its application. AI can improve efficiency by matching manuscripts with appropriate reviewers and flagging issues like conflicts of interest. This can significantly reduce the time it takes to complete peer reviews, ensuring a more efficient process for both authors and reviewers. When applied thoughtfully, these advancements can bridge gaps by creating a smoother and more standardized review process.
However, there is also the risk that researchers from more resource-limited areas could be left behind13 because they might not have access to the necessary tools and infrastructure. Access to reliable internet and advanced tools can be limited in resource-constrained settings, creating disparities. To ensure fairness, platforms must be designed to accommodate different regions and expertise levels, fostering inclusivity and global collaboration in the academic community.
Virtual Peer Review Platforms: Convenience or Complexity?
Virtual peer review platforms are online systems that facilitate the peer review process, allowing reviewers, editors, and authors to interact, submit, and evaluate manuscripts in a digital environment. In their 2 decades of use, virtual peer review platforms have certainly brought convenience to the review process, offering benefits like global accessibility, streamlined workflows, and faster submissions. However, as these platforms have expanded and grown, they have introduced new challenges. For example, one main concern is the potential depersonalization of the review process as interactions become more automated and less personal. Reviewers often face fatigue because of the overwhelming number of requests through these platforms.10 To maintain the human connection, it is essential to encourage personalized feedback and create open peer review systems in which authors and reviewers can collaborate more closely.
Although virtual platforms have made it easier to handle a large volume of manuscripts, they also create a steep learning curve for reviewers and editors transitioning from traditional methods. The impersonal nature of automated notifications can make it difficult for reviewers to feel connected to the work. Despite these complexities, technology, including AI, has improved the efficiency of tasks such as plagiarism detection and reviewer selection. Moving forward, incorporating innovations like interactive manuscript formats and better incentives for reviewers could help address some of these challenges by balancing convenience with a more personal, human approach to peer review.
Human–AI Collaboration in Peer Review: A Partnership or a Power Struggle?
Integrating AI into peer review has sparked debate over whether it should be viewed as a partnership or a power struggle. AI can handle routine tasks like plagiarism detection, statistical checks, and manuscript screening, which allows human reviewers to focus on more complex evaluations, such as ethical considerations and the research’s broader context.1 When AI complements human expertise, it enhances the efficiency and quality of peer review without threatening human judgment.
Still, achieving this balance requires careful implementation. Human reviewers bring irreplaceable insights, especially in areas like ethics, critical thinking, and understanding subtle research nuances. Whereas AI can assist in repetitive tasks, human oversight remains essential to ensure the technology is used responsibly. The future of peer review will likely involve deeper collaboration between AI and humans, where AI supports reviewers without replacing their crucial role in maintaining the integrity and quality of the peer review process.
Conclusion
The growing role of AI and technology enhances the peer review process, offering efficiency improvements through tools like automated plagiarism detection and reviewer selection. However, concerns about bias, data security, and the potential loss of the human element remain significant. Experts stress the need for human oversight, as AI cannot replace human reviewers’ critical thinking and ethical judgment. Open peer review and virtual platforms are acknowledged for their transparency but present challenges such as depersonalization and the risks to professional relationships. While these innovations offer benefits, their widespread adoption, particularly in high-impact journals, could be hindered by reputation and career advancement concerns.
The key takeaway is that the future of peer review requires a balanced approach, integrating AI with human expertise. Transparent guidelines, responsible AI use, and a focus on inclusivity will be essential for building a more equitable, efficient, and reliable peer review system.
Acknowledgments
The authors extend their gratitude to the ACSE for organizing an engaging interview series during Peer Review Week 2024. The series provided a platform for real-time brainstorming on the challenges facing peer review and explored potential solutions to enhance its effectiveness in the future.
Disclosures and Author Contributions
The authors declare no conflicts of interest. The authors received no grants from commercial, governmental, or non-profit organizations related to this work. The opinions expressed in this article are the authors’ personal views and do not represent those of their affiliated organizations, employers, or associations. Muhammad Sarwar: Editing, Review; Maria Machado: Writing, Editing, Proofreading; Jeffrey Robens: Writing, Editing, Proofreading; Gareth Dyke: Writing, Editing, Review; Maryam Sayab: Conceived the Idea, Initial Drafting, Editing, and Review
References and Links
- Kadri SM, Dorri N, Osaiweran M, Garyali P, Petkovic M. Scientific peer review in an era of artificial intelligence. In: Joshi PB, Churi PP, Pandey M, editors. Scientific publishing ecosystem. Springer Nature Singapore; 2024. pp. 397–413. https://doi.org/10.1007/978-981-97-4060-4_23.
- Ross-Hellauer T. What is open peer review? A systematic review. F1000Research. 2017;6:588. https://doi.org/10.12688/f1000research.11369.2.
- [JMIR] Journal of Medical Internet Research Editorial Director. What is open peer review? [accessed December 4, 2024]. https://support.jmir.org/hc/en-us/articles/115001908868-What-is-open-peer-review.
- Pros and cons of open peer review. Nat Neurosci. 1999;2(3):197–198. https://doi.org/10.1038/6295.
- Reinhart M, Schendzielorz C. Peer-review procedures as practice, decision, and governance—the road to theories of peer review. Sci Publ Policy. 2024;51:543–552. https://doi.org/10.1093/scipol/scad089.
- Bell K, Kingori P, Mills D. Scholarly publishing, boundary processes, and the problem of fake peer reviews. Sci Technol Human Values. 2024;49:78–104. https://doi.org/10.1177/01622439221112463.
- Shrivastava A. The role of artificial intelligence in enhancing public policy. AIM Research. [accessed December 4, 2024]. https://aimresearch.co/council-posts/the-role-of-artificial-intelligence-in-enhancing-public-policy.
- Chaka C. Reviewing the performance of AI detection tools in differentiating between AI-generated and human-written texts: a literature and integrative hybrid review. J Appl Learn Teach. 2024;7. https://doi.org/10.37074/jalt.2024.7.1.14.
- Ball R, Talal AH, Dang O, Muñoz M, Markatou M. Trust but verify: lessons learned for the application of AI to case-based clinical decision-making from postmarketing drug safety assessment at the US Food and Drug Administration. J Med Internet Res. 2024;26:e50274. https://doi.org/10.2196/50274.
- Hosseini M, Horbach SP. Fighting reviewer fatigue or amplifying bias? Considerations and recommendations for use of ChatGPT and other large language models in scholarly peer review. Res Integr Peer Rev. 2023;8:4. https://doi.org/10.1186/s41073-023-00133-5.
- Kousha K, Thelwall M. Artificial intelligence to support publishing and peer review: a summary and review. Learned Publ. 2024;37:4–12. https://doi.org/10.1002/leap.1570.
- Kuznetsov I, Afzal OM, Dercksen K, Dycke N, Goldberg A, Hope T, Hovy D, Kummerfeld JK, Lauscher A, Leyton-Brown K, et al. What can natural language processing do for peer review? arXiv:2405.06563. https://doi.org/10.48550/arXiv.2405.06563.
- Pike A, Béal V, Cauchi-Duval N, Franklin R, Kinossian N, Lang T, Leibert T, MacKinnon D, Rousseau M, Royer J, et al. ‘Left behind places’: a geographical etymology. Regional Studies. 2024;58:1167–1179. https://doi.org/10.1080/00343404.2023.2167972.
Muhammad Sarwar (https://orcid.org/0000-0001-9537-2541) is with the Asian Council of Science Editors, Dubai, UAE; Maria Machado (https://orcid.org/0000-0002-2729-4809) is with Storytelling for Science, Porto, Portugal; Jeffrey Robens (https://orcid.org/0000-0003-2344-0036) is with Nature Portfolio, Tokyo, Japan; Gareth Dyke (https://orcid.org/0000-0002-8390-7817) is with Reviewer Credits, Berlin, Germany; and Maryam Sayab (https://orcid.org/0000-0001-7695-057X; maryamsayab@theacse.com) is with Asian Council of Science Editors, Dubai, UAE.
Opinions expressed are those of the authors and do not necessarily reflect the opinions or policies of their employers, the Council of Science Editors, or the Editorial Board of Science Editor.